Detector BYOD: COCO Format Example
Bring Your Own COCO Formatted Data
Understanding the Ingestor File
Let's begin with the MS COCO format ingestor. Find the MS COCO-like dataset template file in the docker container located at /latentai/custom-configs/data/coco-like-template.yaml
It will look like this:
defaults:
- /data/transforms@module.train_transforms: resize.yaml
- /data/transforms@module.valid_transforms: resize.yaml
- _self_
nclasses: 0 # number of classes in your detection dataset
module:
_target_: af.core.data.modules.adaptermodule.AdaptorDataModule
batch_sizes: ${task.batch_sizes}
num_workers: ${task.num_workers}
adaptors: ${model.adaptors}
dataset_generator:
_target_: af.core.data.generic.coco_like.COCOlike
root_path: null # full path to your dataset
download_from_url: null
task_family: detection
train_images_dir: train # path to folder containing training images, relative to root_path
val_images_dir: validation # path to folder containing only validation images, relative to root_path
train_annotations_json: annotations/instances_train.json # path to .json file of training annotations, relative to root_path
val_annotations_json: annotations/instances_val.json # path to .json file of validation annotations, relative to root_path
label_indexing: 1-indexed-no-background # one of: "0-indexed-no-background", "1-indexed-no-background", "0-indexed-with-background". if dataset contains a background class, it must be at index 0.
dataset_name: my-custom-coco-like-data # string - any name for your data
The fields you will need to change are for the number of classes in your dataset and the dataset_generator
fields shown here from line 14 on. Changing those fields with your dataset’s information is enough to load and use your COCO-formatted dataset.
nclasses
: number of classes in your datasetroot_path
: absolute path to the root directory of the datasetdownload_from_url
: if your data is compressed to a single file, and resides in the cloud (public S3 bucket, Drive, etc.) the ingestor can download it and place it insideroot_path
task_family
: detection or segmentation (COCO annotations supports both)train_images_dir
: path to folder containing only training images, relative to root_pathval_images_dir
: path to folder containing only validation images, relative to root_pathtrain_annotations_json
: path to .json file of training annotations, relative to root_pathval_annotations_json
: path to .json file of validation annotations, relative to root_pathlabel_indexing
: Are the labels 0 indexed or is the first class at index 1? Is there a background class? One of0-indexed-no-background
,1-indexed-no-background
,0-indexed-with-background.
The AF needs this information to decide if it needs to label shift to ensure that if a background class is present it must be at index 0, and other classes start at index 1.dataset_name
: a string. Will be used to name any generated artifacts.
By default, everything except the root_path
is set to match the COCO dataset defaults. The folder structure is expected to be:
path/to/mydataset/
|---train
|---image1.jpeg
|---image2.jpeg
...
|---validation
|---image8.jpeg
|---image9.jpeg
...
|---annotations
|---instances_train.json
|---instances_val.json
Now that you have customized the YAML file for your dataset, you can use it as a component by passing its name to the data
parameter, like this:
af --config-name=yolov5_S_RT data=coco-like-template \
command=train task.moniker="BYOD_recipe"
A Concrete BYOD COCO-like Example
SODA10M Dataset Overview
Let’s look at the SODA10M dataset as an example. From the website, we can see that the folder structure does not exactly match the LEIP Recipe defaults:
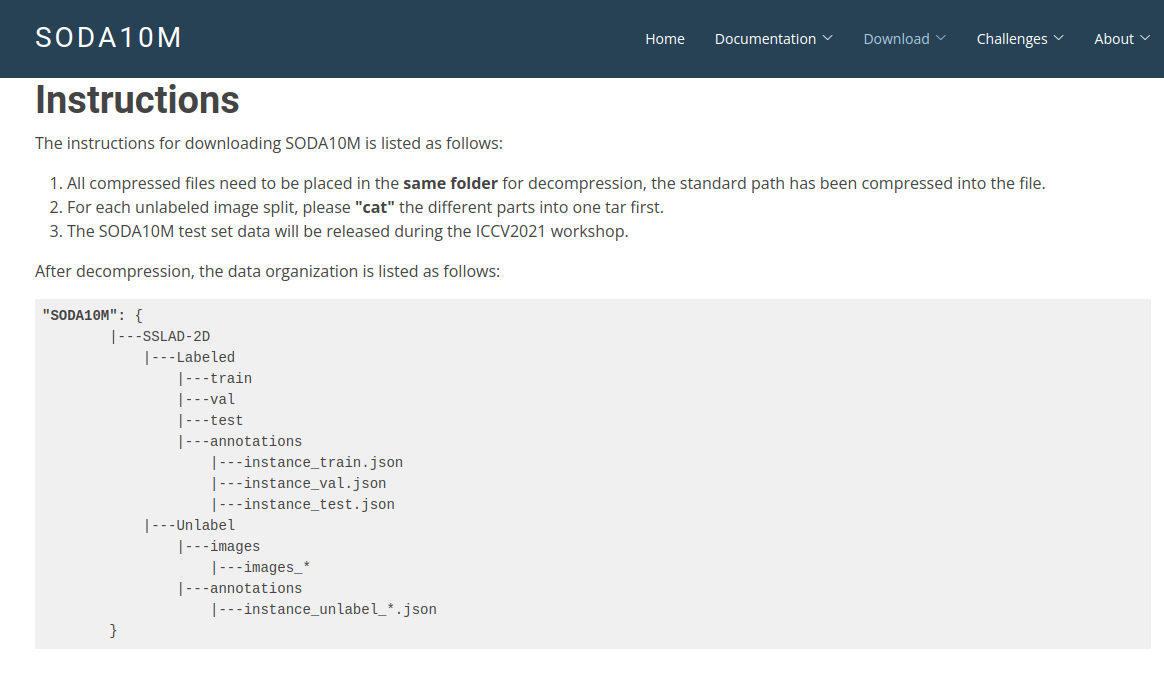
Download the SODA10 labeled dataset from the website. You will download the following two files: labeled_train.tar
and labeled_test.tar
. Create a /latentai/soda10m
directory in the SDK container, copy those files there, and then untar them. Verify that the data has been located in the proper directory:
# Verify data location matches the directories in this example:
ls /latentai/soda10m/SSLAD-2D/labeled/
annotations test train val
Notice the slight difference in annotation files: their instance file, which should now reside in /latentai/soda10m/SSLAD/labeled/annotations
, uses instance_train.json
instead of the plural instances_train.json
. Dont bother renaming files. Rather, see below how to use the YAML configuration to account for this detail.
Using the Template to Ingest the SODA10M Data
Now we need to create a YAML configuration file for this dataset. Create a new file custom-configs/data/soda10m_config.yaml
by copying our template:
cp /latentai/custom-configs/data/coco-like-template.yaml \
/latentai/custom-configs/data/soda10m_config.yaml
Then, modify the fields in the file soda10m_config.yaml
to match the needs of this dataset.
The soda10m_config.yaml
will look like this:
defaults:
- /data/transforms@module.train_transforms: resize.yaml
- /data/transforms@module.valid_transforms: resize.yaml
- _self_
nclasses: 6
module:
_target_: af.core.data.modules.adaptermodule.AdaptorDataModule
batch_sizes: ${task.batch_sizes}
num_workers: ${task.num_workers}
adaptors: ${model.adaptors}
dataset_generator:
_target_: af.core.data.generic.coco_like.COCOlike
root_path: /latentai/soda10m/SSLAD-2D/labeled
download_from_url: null
task_family: detection
val_images_dir: val
train_images_dir: train
train_annotations_json: annotations/instance_train.json
val_annotations_json: annotations/instance_val.json
label_indexing: 1-indexed-no-background
dataset_name: soda10m
Train
You can now train on this dataset by running the following:
af --config-name=yolov5_S_RT data=soda10m_config \
command=train task.moniker="BYOD_recipe"
Understanding the command:
--config-name=yolov5_L_RT
: select theyolov5_L_RT
recipedata=soda10m_config
: use thedata/soda10m_config.yaml
file as the data config for this recipeIt's important to ensure the
.yaml
is nested inside an additionaldata
folder withincustom-configs
task.moniker="BYOD_recipe"
Gives this run a different name, so we don't confuse its artifacts with the pretrained model’s artifacts you generated in the previous sections.
Note where the checkpoint is stored at the end of the training run. The checkpoint will be stored in a path of the form:/latentai/artifacts/train/{date}_{time}_BYOD_recipe/{epoch}.ckpt
Find that file and store it for ease of use in the next steps:
export SODA_CHECK=<path to .ckpt file>
# Example:
# export SODA_CHECK=/latentai/artifacts/train/2022-08-23_17-25-29_task_BYOD_recipe/epoch-19_step-1840.ckpt
Note: the default recipe parameters are not expected to yield optimal training results for this dataset. This tutorial is only meant to show how to ingest COCO formatted data. Please refer to Advanced AF Options for instructions on how to tune hyperparameters.
Evaluate on the Host Device
You can now use your newly trained model by passing the checkpoint to the commands you used previously with the pre-trained model. For example, to evaluate:
af --config-name=yolov5_S_RT data=soda10m_config \
command=evaluate task.moniker="BYOD_recipe" \
+checkpoint=$SODA_CHECK
Visualize the Predictions on Host Device
The AF command=predict
allows you to predict on a few samples of the data using the trained model checkpoint.
af --config-name=yolov5_S_RT data=soda10m_config \
command=predict task.moniker="BYOD_recipe" \
+checkpoint=$SODA_CHECK \
model.module.score_thresh=0.4
Understanding the command:
model.module.score_thresh=0.4
: Only visualize samples that have a confidence score > 40 percent. This is necessary to avoid cluttering the visualization with too many boxes. Feel free to play with this number. A lower value will display more boxes, a higher value will display less boxes.
The predicted images will be stored in /latentai/artifacts/predictions/soda10m/*
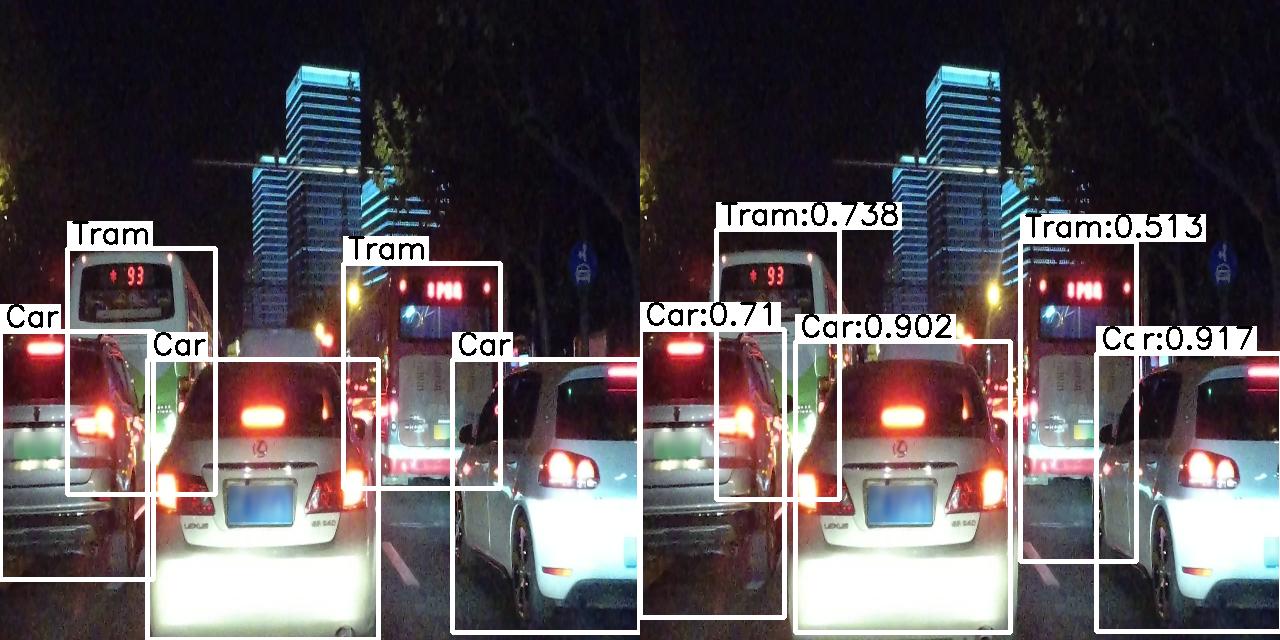
Left: Ground truth example from the validation set.
Right: Predictions generated by the trained checkpoint.
Export the Model to be Ingested by the LEIP SDK
af --config-name=yolov5_S_RT data=soda10m_config \
command=export task.moniker="BYOD_recipe" \
+checkpoint=$SODA_CHECK
Your exported model will be saved in:/latentai/artifacts/export/BYOD_recipe_batch1_640-640/traced_model.pt
Evaluating the SODA10 Example on the Target
Before you can optimize your trained model, you need to identify a calibration dataset from your BYOD data. Identifying an ideal calibration dataset is beyond the scope of this tutorial, but usually entails selecting a few representative images for the quantizer. If you are targeting a CUDA device, you will need to provide 2N images, where N is your batch size. You will need to provide a path to these images in the file rep_dataset.txt
that is in the model recipe directory. We will choose four images in the validation dataset for the SODA10 dataset.
For Yolov5 Small, the representative dataset file is /latentai/recipes/yolov5_S_RT/rep_dataset.txt
. The default rep_dataset has been chosen for MSCOCO optimization. Copy this file to a safe place, and change the content of the file to contain the following paths to images in the SODA10 dataset:
/latentai/soda10m/SSLAD-2D/labeled/val/HT_VAL_004979_SH_001.jpg
/latentai/soda10m/SSLAD-2D/labeled/val/HT_VAL_004980_SH_010.jpg
/latentai/soda10m/SSLAD-2D/labeled/val/HT_VAL_004981_GZ_231.jpg
/latentai/soda10m/SSLAD-2D/labeled/val/HT_VAL_004982_SH_010.jpg
If you find that your optimized (Int8) model has substantial accuracy loss from the compiled (Float32) model, try different calibration images. Some image sets will produce outlier results that may adversely affect accuracy.
Please bear in mind that the optimal training dataset may change each time you retrain the model. The above is an example dataset, but you may need to test out different datasets to yield the best results after you have trained your model.
If you do not have the utils directory in your PYTHONPATH from Step Two, you will need to set it now before running the leip pipeline
command:export PYTHONPATH=/latentai/recipes/yolov5_L_RT/evaluation/utils:$PYTHONPATH
Once you have exported your trained model and determined a calibration dataset, simply compile and optimize it as before, providing the traced model as the input path:
leip pipeline \
--input_path /latentai/artifacts/export/BYOD_recipe_batch1_640-640/traced_model.pt \
--output_path /latentai/workspace/recipes_output/yolov5_S_RT/x86_64_cuda \
--config_path /latentai/recipes/yolov5_S_RT/pipeline_x86_64_cuda.yaml
If you would like to use leip evaluate
to evaluate your model, you will need to copy one file to prepare the validation dataset:
# Some processes will look for the instances file in the data directory:
cp /latentai/soda10m/SSLAD-2D/labeled/annotations/instance_val2017.json \
/latentai/soda10m/SSLAD-2D/labeled/val/
You can now evaluate your compiled, optimized model against the Smoke dataset:
# Evaluate Float32:
leip evaluate \
--input_path /latentai/workspace/recipes_output/yolov5_S_RT/x86_64_cuda/Float32-compile/ \
--test_path /latentai/soda10m/SSLAD-2D/labeled/val/instance_val.json \
--dataset_type coco --output_format yolov5
# Evaluate Int8:
leip evaluate \
--input_path /latentai/workspace/recipes_output/yolov5_S_RT/x86_64_cuda/Int8-optimize/ \
--test_path /latentai/soda10m/SSLAD-2D/labeled/val/instance_val.json \
--dataset_type coco --output_format yolov5
If you would like to evaluate your BYOD model on the target device, follow these additional steps.